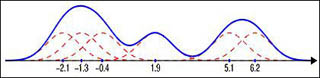
Six Gaussians (red) and their sum (blue). The Parzen window density estimate f(x) is obtained by dividing this sum by 6, the number of Gaussians. The variance of the Gaussians was set to 0.5. Note that where the points are denser the density estimate will have higher values.
Instructor(s)
Media Lab Faculty and Staff
MIT Course Number
MAS.622J / 1.126J
As Taught In
Fall 2006
Level
Graduate
Course Description
Course Features
Course Description
This class deals with the fundamentals of characterizing and recognizing patterns and features of interest in numerical data. We discuss the basic tools and theory for signal understanding problems with applications to user modeling, affect recognition, speech recognition and understanding, computer vision, physiological analysis, and more. We also cover decision theory, statistical classification, maximum likelihood and Bayesian estimation, nonparametric methods, unsupervised learning and clustering. Additional topics on machine and human learning from active research are also talked about in the class.